The integration of artificial intelligence (AI) and machine learning (ML) into stealth and radar technologies represents a key element of the race to the top of defense technologies currently taking place. These offensive and defensive capabilities are constantly evolving with AI/ML serving as the next step in their evolution.
Integrating AI/ML into low-observable technology presents a promising avenue for enhancing stealth capabilities, but it also comes with its own set of challenges. ML algorithms rely on large volumes of high-quality data for training and validation. Acquiring such data for low-observable technology is challenging due to the classified nature of military operations and the limited availability of real-world stealth measurements.
ML algorithms analyze vast amounts of radar data to identify patterns and anomalies that were previously undetectable. This includes the ability to track stealth aircraft and missiles with greater accuracy and speed. These advancements have significant implications for deterrence strategies as traditional stealth technology may diminish in its effectiveness as AI/ML-powered radar becomes more sophisticated, potentially undermining the deterrent value of stealth aircraft and missiles.
Stealth technology remains a cornerstone of deterrence, allowing military assets to operate relatively undetected. Radar, on the other hand, is the primary tool for detecting and tracking these assets. However, AI/ML are propelling both technologies into new frontiers. AI algorithms can now design and optimize stealth configurations that were previously impossible. This includes the development of adaptive camouflage that dynamically responds to changing environments, making detection even more challenging.
Furthermore, stealth technology encompasses a multitude of intricately designed principles and trade-offs, including radar cross-section (RCS) reduction, infrared signature management, and reduction of acoustic variables. Developing ML algorithms capable of comprehensively modeling and optimizing these complex interactions poses a significant challenge. Moreover, translating theoretical stealth concepts into practical design solutions that can be effectively learned by ML models requires specialized domain knowledge and expertise.
As ML-based stealth design techniques become more prevalent, adversaries may employ adversarial ML strategies to exploit vulnerabilities and circumvent the defenses afforded to stealth aircraft. Adversarial attacks involve deliberately perturbing input data to deceive ML models and undermine their performance. Mitigating these threats requires the development of robust countermeasures and adversarial training techniques to enhance the resilience of ML-based stealth systems.
Additional complexities are inherent in the fact that ML algorithms often operate as “black boxes,” making it challenging to interpret their decision-making processes and understand the underlying rationale behind their predictions. In the context of stealth technology, where design decisions have significant operational implications, the lack of interpretability and explainability poses a barrier to trust and acceptance. Ensuring transparency and interpretability in ML-based stealth design methodologies is essential for fostering confidence among stakeholders and facilitating informed decision-making.
Implementing ML algorithms for stealth optimization involves computationally intensive tasks, including data preprocessing, model training, and simulation-based optimization. As low-observable technology evolves to encompass increasingly sophisticated designs and multi-domain considerations, the computational demands of ML-based approaches may escalate exponentially. Balancing computational efficiency with modeling accuracy and scalability is essential for practical deployment in real-world military applications.
Integrating AI and ML into military systems raises complex regulatory and ethical considerations, particularly regarding autonomy, accountability, and compliance with international laws and conventions. Ensuring that ML-based stealth technologies adhere to ethical principles, respect human rights, and comply with legal frameworks governing armed conflict is paramount. Moreover, establishing transparent governance mechanisms and robust oversight frameworks is essential to addressing concerns related to the responsible use of AI in military applications.
Addressing these challenges requires a concerted interdisciplinary effort, bringing together expertise from diverse fields such as aerospace engineering, computer science, data science, and ethics. By overcoming these obstacles, AI/ML has the potential to revolutionize low-observable technology, enhancing the stealth capabilities of military aircraft and ensuring their effectiveness in an increasingly contested operational environment. On the other hand, AI/ML has the potential to significantly impact radar technology, posing challenges to conventional low-observable and stealth aircraft designs in the future.
AI/ML algorithms can enhance radar signal processing capabilities by improving target detection, tracking, and classification in cluttered environments. Analyzing complex radar returns and discerning subtle patterns indicative of stealth aircraft, these algorithms can mitigate the challenges posed by low-observable technology, making it more difficult for stealth aircraft to evade detection.
ML algorithms can optimize radar waveforms in real time based on environmental conditions, target characteristics, and mission objectives. Dynamically adjusting waveform parameters such as frequency, amplitude, and modulation, radar systems can exploit vulnerabilities in stealth designs—increasing the probability of detection. This adaptive approach enhances radar performance against evolving threats, including stealth aircraft with sophisticated countermeasures.
Cognitive radar systems leverage AI/ML techniques to autonomously adapt their operation and behavior in response to changing operational environments. These systems learn from past experiences, anticipate future scenarios, and optimize radar performance adaptively. Continuously evolving their tactics and strategies, cognitive radar systems can outmaneuver stealth aircraft and exploit weaknesses in their low-observable characteristics.
AI/ML facilitates the coordination and synchronization of multi-static and distributed radar networks, comprising diverse sensors deployed across different platforms and locations. By fusing information from multiple radar sources and exploiting the principles of spatial diversity, these networks can enhance target detection and localization capabilities. This collaborative approach enables radar systems to overcome the limitations of individual sensors and effectively detect stealth aircraft operating in contested environments.
ML techniques can be employed to develop countermeasures against stealth technology by identifying vulnerabilities and crafting effective detection strategies. By generating adversarial examples and training radar systems to recognize subtle cues indicative of stealth aircraft, researchers can develop robust detection algorithms capable of outperforming traditional radar techniques. ML provides a proactive defense mechanism against stealth threats, potentially rendering conventional low-observable technology obsolete.
AI and ML enable the construction of data-driven models and simulations that accurately capture the electromagnetic signatures and propagation phenomena associated with stealth aircraft. By leveraging large datasets comprising radar measurements, electromagnetic simulations, and physical modeling, researchers can develop comprehensive models of stealth characteristics and devise innovative counter-detection strategies. These data-driven approaches provide valuable insights into the vulnerabilities of stealth technology and inform the design of more effective radar systems.
In the quest for technological superiority in modern warfare, the integration of AI and ML into radar technology holds significant promise with the potential to challenge conventional low-observable and stealth aircraft designs by enhancing radar-detection capabilities. AI and ML algorithms improve radar signal processing, optimize radar waveforms in real time, and enable radar systems to autonomously adapt their operation. By leveraging multi-static and distributed radar networks and employing adversarial ML techniques, researchers can develop robust detection algorithms capable of outperforming traditional radar systems. Moreover, data-driven modeling and simulation provide insights into the vulnerabilities of stealth technology, informing the design of more effective radar systems.
The rapid advancement of AI/ML is revolutionizing both stealth and radar technologies, with profound implications for deterrence strategies. Traditionally, deterrence has relied on the balance of power and the credible threat of retaliation. However, the integration of AI/ML into these technologies is fundamentally altering the dynamics of detection, evasion, and response, thereby challenging the established tenets of deterrence. Of further concern is the consideration that non-stealth assets become increasingly vulnerable to detection and targeting as ML-powered radar systems become more prevalent. This could lead to a greater reliance on stealth technology, further accelerating the arms race.
This rapid development of AI/ML-powered technologies could destabilize the existing balance of power, leading to heightened tensions and miscalculations. The changing technological landscape may necessitate the development of new deterrence strategies that incorporate AI and ML. This could include a greater emphasis on cyber warfare and the development of counter-AI and counter-ML capabilities.
The integration of AI/ML into stealth and radar technologies will be a game-changer for deterrence. To maintain stability and prevent conflict, policymakers and military strategists must adapt to this new reality of a continuous arms race, wherein both offensive and defensive capabilities are constantly evolving in pursuit of technological superiority. Continued investment in AI/ML research is essential to stay ahead of the curve and maintain a credible deterrent posture. International cooperation on the development and use of AI/ML technologies in military applications is crucial to limit the scope of a potential arms race that regularly shifts the balance of power and destabilizes global security.
Joshua Thibert is a Contributing Senior Analyst at the National Institute for Deterrence Studies (NIDS) and doctoral candidate at Missouri State University. His extensive academic and practitioner experience spans strategic intelligence, multiple domains within defense and strategic studies, and critical infrastructure protection. The views expressed in this article are the author’s own
About the Author
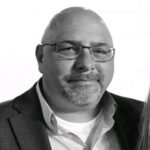
Joshua Thibert
Joshua Thibert is a Contributing Senior Analyst at the National Institute for Deterrence Studies (NIDS)with over 30 years of comprehensive expertise, his background encompasses roles as a former counterintelligence special agent within the Department of Defense and as a practitioner in compliance, security, and risk management in the private sector. His extensive academic and practitioner experience spans strategic intelligence, multiple domains within defense and strategic studies, and critical infrastructure protection.
There is no doubt that the Regime will ue AI against its political opponent.